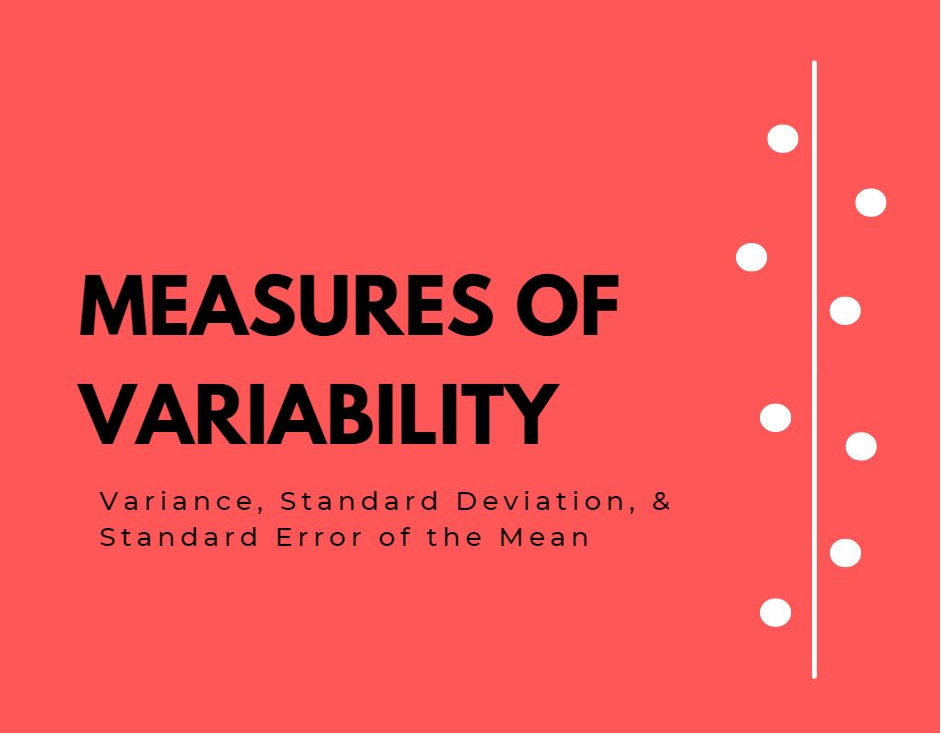
Table of Contents
What are Measures of Variability?
In previous articles we have learned that applying descriptive statistics of central tendency involves considering the frequency distribution of a dataset and then computing the appropriate measure of central tendency (e.g., mean and median). This information simplifies the data distribution and allows you to envision its general properties.
But we need more information to have a complete description of any set of data. Thus, we need to measure its variability to know how much the individual scores differ from each other and from the center and how spread out the distribution of observation is.
Note from the Table 1 that the three datasets (A, B and C) have the same mean (x̄ = 6) with different distributions. The mean tells us nothing about variation or how data are spread out from each other and from their mean. Therefore, we need to use the measures of variability such as variance, standard deviation and standard error of the mean.
Table 1: Three datasets with same mean but differ in variability
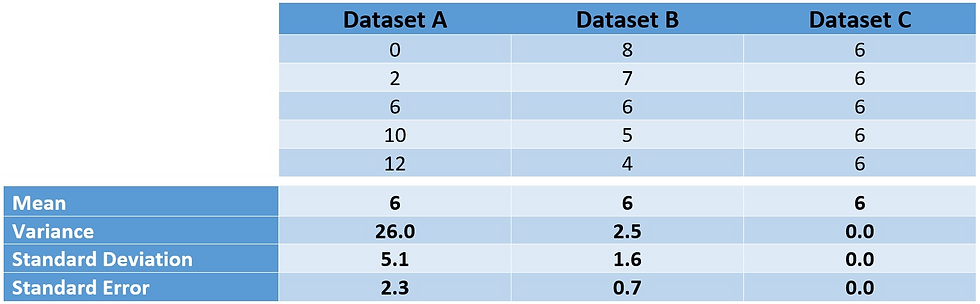
Notice that from the three datasets, the closer the scores are to each other and to the mean, the smaller the variability (variance, standard deviation and standard error).
Variance
Mathematically, the distance between a score and the mean (ȳ) is the difference between them. The variance is the average of the squared differences between each data point and the mean of the data set and The larger the variance, the more spread out the data is.
The variance can be computed using the following formulas for variance of a population (σ²) and a sample (s²):
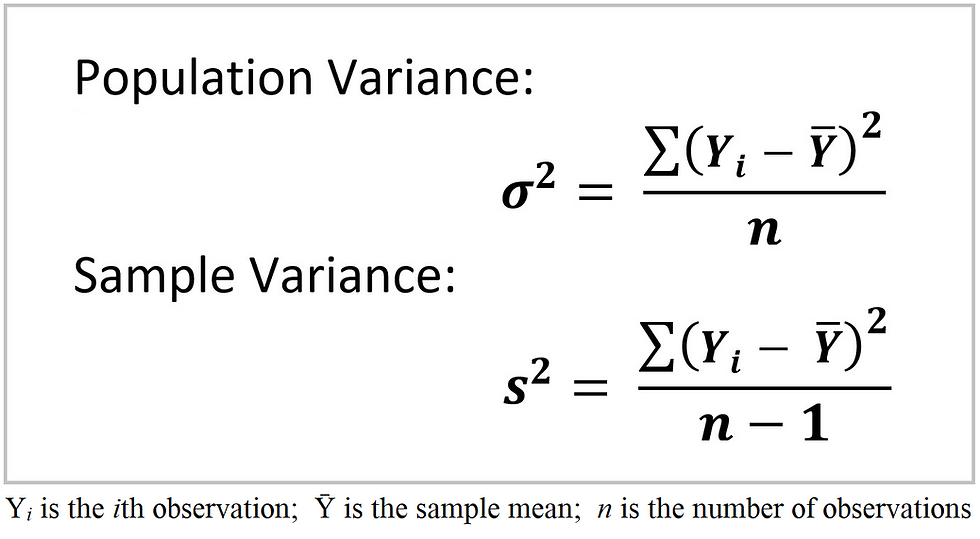
The variance has different units than the data for which it was calculated, so it is not very intuitive to interpret. For example, if the data were measured in inches, the variance would be measured in square inches. Therefore, it is often more useful to look at the standard deviation, which is the square root of the variance and has the same unit as the data points.
Standard Deviation
The standard deviation is a measure of the spread of a data set, similar to the variance. It is defined as the square root of the variance of a data set as shown in the following formulas:
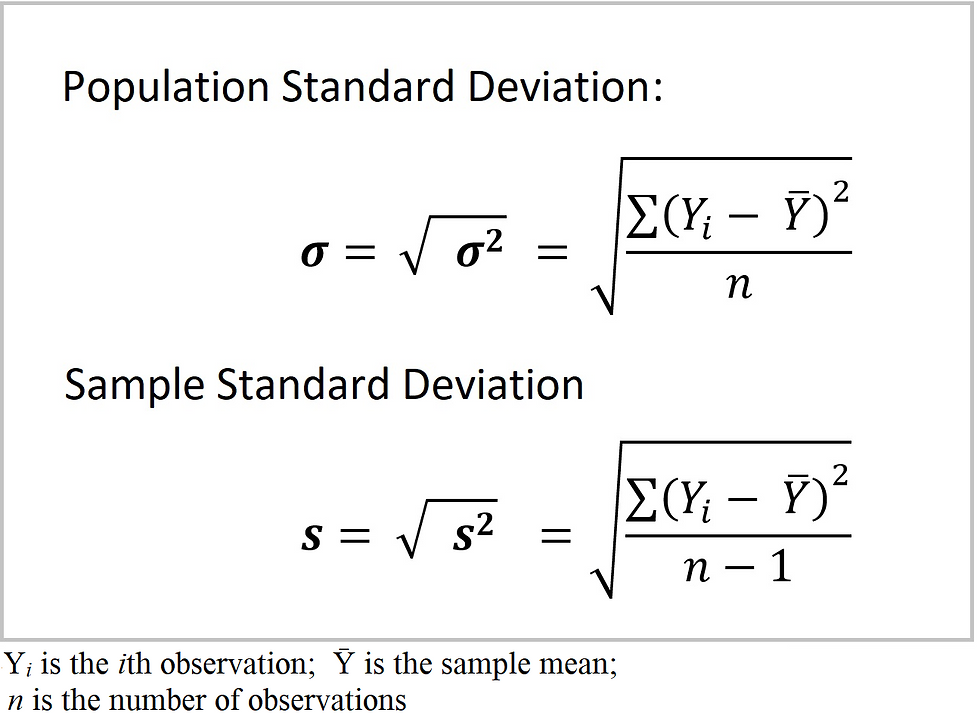
The standard deviation has the same unit as the data, which makes it more intuitive to interpret than the variance. For example, if the data set represents a population's weight in pounds, the standard deviation will also be in pounds.
The standard deviation is also used to calculate the standard error of the mean (SEM) which is a measure of the precision of the sample mean.
Standard Error of the Mean
The standard error of the mean (SEM) can be thought of as the average amount that the sample means (ȳ) deviate from the actual population mean (μ) and can be calculated by dividing the standard deviation by the square root of n, where n is the number of observations, as shown in the following formula:
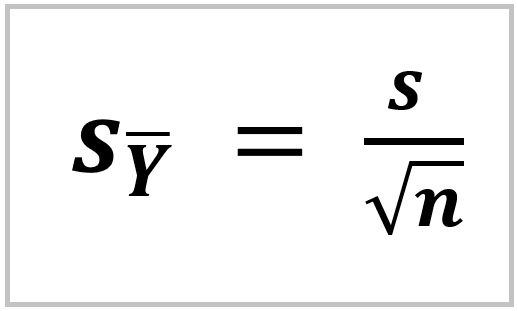
The Standard error value gets smaller as the sample size gets larger, because a larger sample size gives a more precise estimate of the population mean.
Example
Suppose we have of a data set contains a 16 patients’ height (8 males and 8 females) (Table 2). We need to know if our sample patients are in the range of the expected healthy average, since the expected mean height of a healthy population should be about 163 cm for women and 176.5 cm for men.
Table 2: Sample data of adults’ males and females heights
Males' height (cm) | Females height (cm) |
172 | 166 |
168 | 162 |
174 | 154 |
175 | 160 |
165 | 148 |
178 | 155 |
163 | 170 |
172 | 163 |
Now, we need to explore the data using basic descriptive statistics to understand our data and draw a conclusion based on this descriptive summary. Many software and programming languages can calculate descriptive statistics for you easily. Here, we used SPSS to get the following results (Table 3).
Table 3: SPSS descriptive results

Descriptive statistics results presented in Table 3 showed that the mean height of males are higher than females. In case we want to know whether this difference is statistically significant or not, we need to use student’s t-test.
The results also showed that the standard deviation of males’ height scores is lower than females’ height scores; the males' height scores are closer to each other (lower variability) than females. In addition, standard error of males data is also lower and consequently males' data is slightly more representative to their population than females.
References
Heiman, G. W. (2011). Basic Statistics for the Behavioral Sciences (6th ed.). USA: Cengage Learning.
Mendenhall, W. M., & Sincich, T. L. (2016). Statistics for Engineering and the Sciences Student Solutions Manual (6th ed.). USA: Taylor & Francis Group, LLC.
Samuels, M. L., Witmer, J. A., & Schaffner, A. (2012). Statistics for the Life Sciences (4th ed.): Pearson Education, Inc.
Weiss, N. A., & Weiss, C. A. (2012). Introductory Statistics (9th ed.): Pearson Education, Inc.
Comments