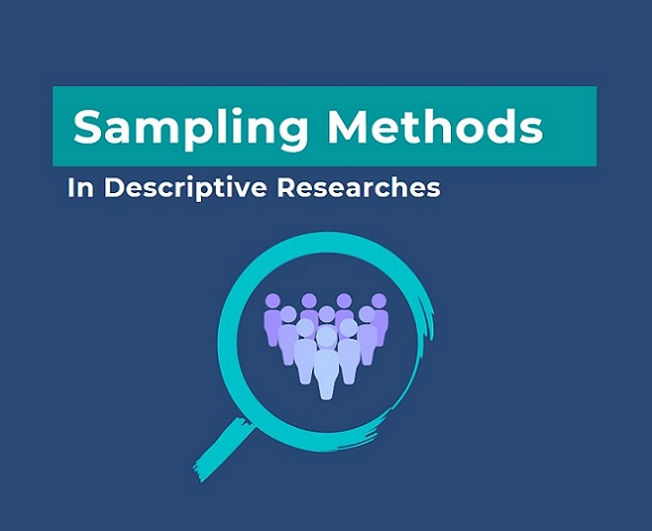
Table of Contents
What is sampling
Any researcher who conducts a descriptive study wants to determine the nature of how things are. Especially when conducting survey research, the researcher may want to describe one or more characteristics of a fairly large population and make inferences about the population.
In such situations, researchers often do not study the entire population of interest. Instead, they select a subset, or sample, of the population and use the results obtained from their sample to make generalizations about the entire population.
Sampling is the process of selecting a portion or subset of a larger group, called the population (Figure 1). The goal of sampling is to gain information about the population and draw inferences about the population based on the characteristics of the sample, and to generalize the findings from the sample to the population.
The key to successful sampling is to ensure that the sample is representative of the population by reducing the risk of bias, so that the inferences drawn from the sample are accurate and reliable. This can be achieved through the use of appropriate sampling methods.
Sampling bias refers to a systematic error in which certain individuals or elements within a population have an increased probability of being selected in a sample, leading to a sample that is not representative of the entire population.
Sampling is widely used in various fields such as statistics, research, quality control, and engineering.
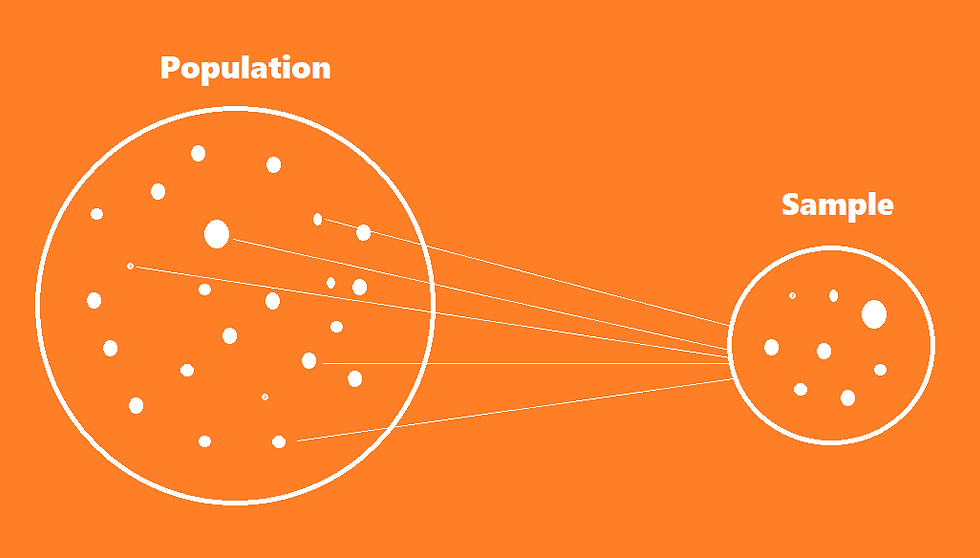
Figure 2: A visual representation of selecting a Sample from a Population
Imagine you are conducting a survey to determine the average household income in a city with a population of 1 million people. To gather this information from every single person in the city would be a daunting and time-consuming task. This is where sampling comes in. By selecting a representative sample of, say, 1000 people from the city, you can estimate the average household income for the entire population based on the data collected from the sample. The results of the sample can then be used to make generalizations about the population as a whole. This is just one example of the need for sampling, as it provides a more efficient and cost-effective way to gather information about a large population.
Sampling Designs
There are different types of sampling methods (designs) and the choice of sampling method depends on the research design, the population characteristics, and the goals of the study. Here we consider eight approaches to sampling, which fall into two major categories: probability sampling and nonprobability sampling.
Probability Sampling
In probability sampling, each member of the population has a known, non-zero chance of being selected for the sample. In other words, it is a random selection process where each member of the population has a known probability of being chosen for the sample.
The main advantage of probability sampling is that it reduces the risk of bias and ensures that the sample is representative of the population, meaning that results from the sample can be generalized to the population as a whole. The most commonly used probability sampling methods include:
Simple Random Sampling
Stratified Random Sampling
Cluster Sampling
1. Simple Random Sampling
In simple random sampling method, every member of the population has an equal chance of being selected. In this method, a sample is selected randomly and independently from the population without any bias or preferential treatment.
The simple random sampling is the most commonly used method because it reduces the risk of selection bias and ensures that the sample is representative of the population.
The process of simple random sampling can be accomplished in various ways, such as using a random number generator, drawing names from a hat, or using a table of random numbers. The sample size can be determined based on the desired level of precision, the size of the population, and other factors.
For example, consider a population of 100 students in a school and you want to select 10 students for a survey. Using simple random sampling, you can generate 10 random numbers between 1 and 100, and then select the students whose number corresponds to the random numbers. This ensures that each student has an equal and independent chance of being selected for the survey.
Simple random sampling is often used when the population is large and homogeneous, and the goal is to make inferences about the population as a whole. However, it may not be the best choice in all situations and other sampling methods, such as stratified random sampling or cluster sampling, may be more appropriate depending on the research design and population characteristics.
2. Stratified Random Sampling
Stratified random sampling is a statistical sampling method where a population is divided into smaller groups (strata) based on certain characteristics, and then a random sample is taken from each stratum. This ensures that each stratum is represented proportionately in the sample, allowing for a more accurate representation of the population as a whole. This method is useful when the population contains subpopulations with different characteristics, and you want to ensure that each subpopulation is represented in the sample.
Stratified random sampling has the advantage of guaranteeing equal representation of each of the identified strata. It is most appropriate when the strata are roughly equal in size in the overall population.
For example, a researcher wants to conduct a study to determine the success rate of a new medical treatment. The study population consists of patients with varying degrees of severity of the condition being treated. You could divide the patients into strata based on the severity of their condition, and then randomly select a sample of patients from each stratum. This way, you ensure that each level of severity is represented proportionately in the sample, and you get a more accurate estimate of the success rate of the treatment for the overall population (Figure 2).
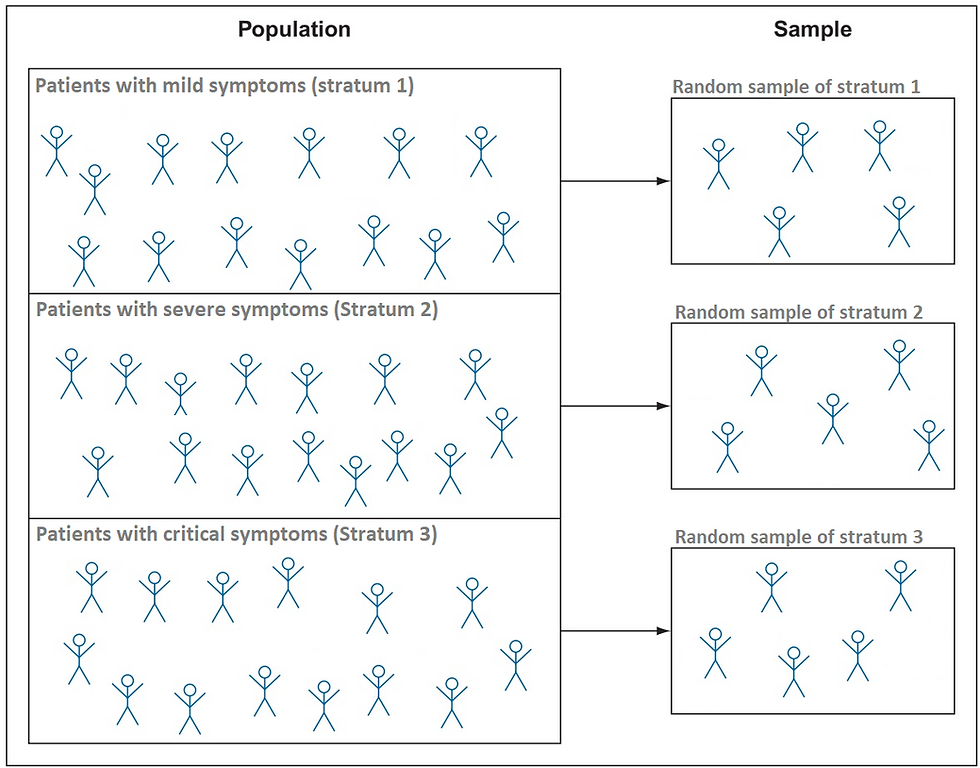
Figure 2: Stratified Random Sampling Design
3. Cluster Sampling
In cluster sampling, the population is divided into multiple groups (clusters), and a sample of clusters are selected using either simple random sampling or stratified random sampling. Then, all units or individuals within each selected cluster are included in the sample.
Imagine you are conducting a survey to determine the average age of students in a university. The university has a large student population, and it is not feasible to survey every student. To accurately represent the university's population, you could use cluster sampling.
You could divide the university into departments (clusters), and randomly select a departments and their students become our sample to participate in the survey (Figure 3). This way, you ensure that each department is represented proportionately in the sample, and you get a more accurate estimate of the average age of students in the university but your sample may not accurately represent the population if one department has a much older or younger student population than the others.
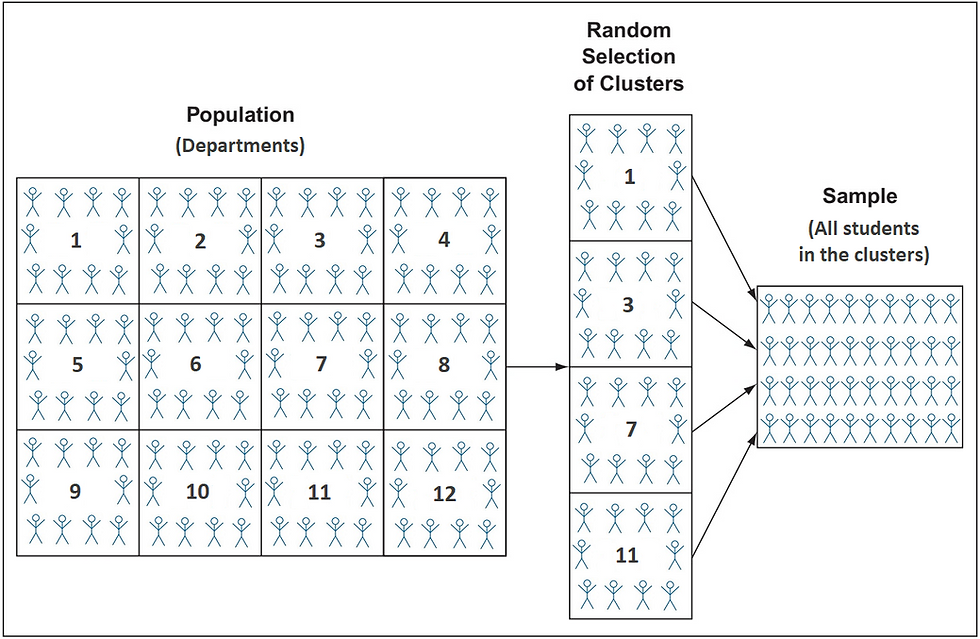
Figure 3: Cluster Sampling Design
Non-probability Sampling
In non-probability sampling, the sample is selected based on convenience or judgment rather than a random process. This type of sampling is less scientifically rigorous because the researcher has no way of predicting or guaranteeing that each element of the population will be represented in the sample. Therefore, the results obtained from the sample cannot be generalized to the population. The most commonly used nonprobability sampling methods include:
Convenience Sampling
Snowball Sampling
Purposive Sampling
1. Convenience Sampling
Convenience sampling—also known as accidental sampling—entails selecting the sample based on ease of access or availability of the subjects. In other words, the sample is chosen simply because they are convenient and readily available.
Convenience sampling can be quick and inexpensive, but the results obtained may not be representative of the population of interest, leading to potential biases and low generalizability of the findings.
2. Snowball Sampling
Snowball sampling (also called network sampling or chain sampling) is a variant of convenience sampling. The process starts by selecting a few initial subjects, and then asking those subjects to refer others from the same population. This continues, with each new subject referring others, until the desired sample size is reached or no more referrals can be made.
This method is often used when the population is difficult to identify and reach. For example, researchers may want to study the experiences of individuals who have overcome addiction and are now in recovery. This population can be challenging to study as many individuals in recovery prefer to keep their experiences private. Therefore, the researchers start by identifying and recruiting a few initial participants through support groups or local treatment centers. Then, they ask these participants to refer others from the same population who they know and trust. The researchers continue to gather referrals in this way until they have reached their desired sample size.
3. Purposive Sampling
In purposive sampling (judgmental sampling), individuals or units are chosen for a particular purpose or characteristics. In other words, the sample units are selected based on certain characteristics (Figure 4) that are deemed relevant to the research question, and the selection process is guided by the researcher's purpose or objective.
This method is commonly used in qualitative research, where the focus is on understanding the experiences, perspectives, and behaviors of individuals in a specific group, rather than making generalizations about the population.
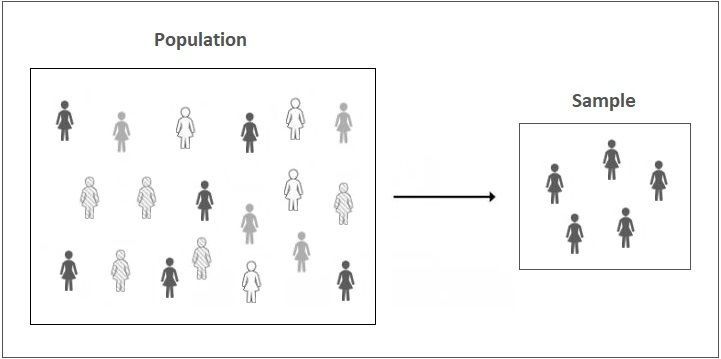
Figure 4: Purposive Sampling
Suppose a researcher is interested in understanding the experiences and perspectives of women who have undergone breast cancer treatment. The researcher could use purposive sampling to select a sample of women who have undergone breast cancer treatment, based on specific criteria such as age, stage of cancer, type of treatment received, etc. The goal is to select a sample of women who are representative of the population of interest and who can provide valuable insights into the experiences and perspectives of women who have undergone breast cancer treatment.
References
Polit, D. F., & Beck, C. T. (2010). Essentials of nursing research: Appraising evidence for nursing practice (7th ed.): Lippincott Williams & Wilkins.
Leedy, P. D., & Ormrod, J. E. (2015). Practical research: Planning and design. USA: Pearson Education.
Beins, B. C., & McCarthy, M. A. (2012). Research methods and statistics. USA: Pearson Education.
Comments