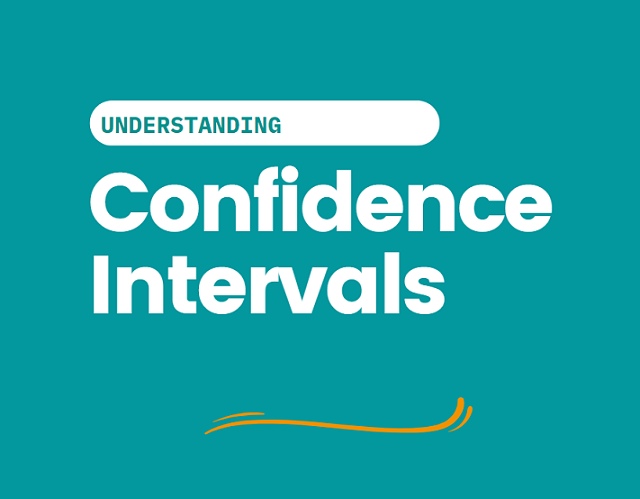
Table of Contents
What is Confidence Interval?
A confidence interval (CI) is a range of values that is likely to contain a population parameter (e.g., mean; μ) with a certain level of confidence.
The confidence level is usually expressed as a percentage (often, 95% or 99%), and it represents the probability that the confidence interval contains the true value of the parameter. For example, a 95% confidence interval means that there is a 95% probability that the interval contains the true value of the parameter.
The width of the confidence interval depends on the sample size and the level of confidence. A larger sample size will result in a narrower confidence interval, and a higher level of confidence will result in a wider interval.
Confidence intervals are commonly used in statistical analysis to estimate population parameters when it is not practical or possible to measure the entire population. They are also useful for understanding the uncertainty associated with estimates of population parameters.
The Importance of Confidence Intervals
Confidence intervals are important because they provide a range of values within which the true value of a population parameter is likely to fall. This is useful because it allows you to make statistical inferences about the population based on a sample, while taking into account the uncertainty inherent in any sample-based estimate.
Using a confidence interval allows you to make a statement about the likely value of the population parameter with a certain level of confidence, rather than just giving a single point estimate that may not accurately reflect the true value. This can be especially useful when the sample size is small or the population is highly variable, as it allows you to quantify the uncertainty associated with your estimate.
For example, suppose you want to know the average income of a certain population. You cannot measure the income of every person in the population, so you take a sample and calculate the average income of the sample. The confidence interval around this estimate tells you how much the true average income of the population is likely to differ from the sample average, based on the sample size and the level of confidence you choose.
An example of Confidence Intervals
Suppose you want to know the average height of adult men in a certain population. You can't measure the height of every man in the population, so you take a random sample of 50 men and calculate their average height (x̄) to be 68 inches. You also calculate the standard deviation of the sample to be 4 inches.
You can use the information above to construct a 95% confidence interval for the population mean height. Using the t-interval method, the confidence interval would be:
68 inches + (2.01 × 4 inches / √50)
This simplifies to:
68 inches + 2.8 inches
So, we are 95% confident that the population mean height lies between [68 - 2.8 = 65.2 inches] and [68 + 2.8 = 70.8 inches]. This means that there is a 95% probability that the true average height of adult men in the population would be from 65.2 to 70.8 inches.
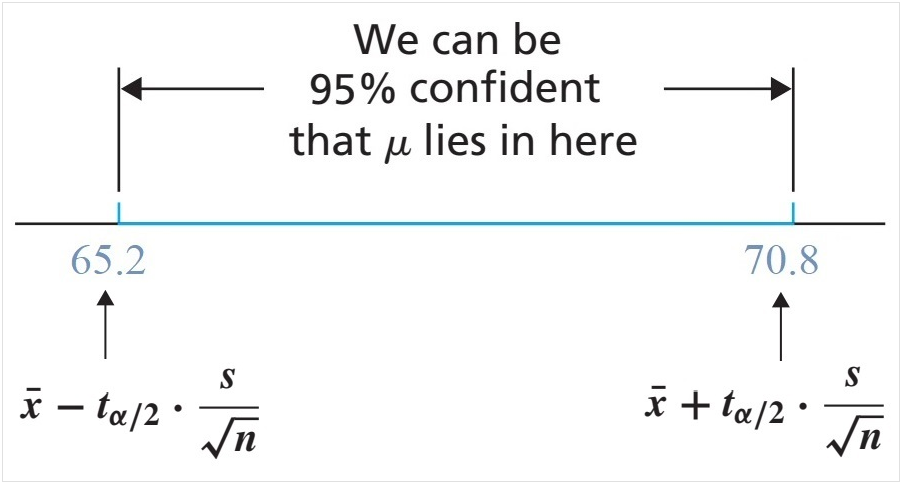
Type of Confidence Intervals
There are several types of confidence intervals that can be used in statistical analysis, including:
One-sample confidence intervals: These intervals estimate a population parameter based on a single sample.
Two-sample confidence intervals: These intervals compare two population parameters based on two samples.
Difference of means confidence intervals: These intervals estimate the difference between two population means based on two samples.
Proportion confidence intervals: These intervals estimate a population proportion based on a sample.
Ratio of means confidence intervals: These intervals estimate the ratio of two population means based on two samples.
Difference of proportions confidence intervals: These intervals estimate the difference between two population proportions based on two samples.
Predictive intervals: These intervals estimate the likely value of a future observation based on a statistical model and a sample.
Tolerance intervals: These intervals estimate the range within which a certain percentage of the population values are likely to fall.
There are also different methods for constructing confidence intervals, including the t-interval, z-interval, and bootstrapped intervals. The appropriate type of interval and method will depend on the specific characteristics of the data and the research question being addressed.
Comments