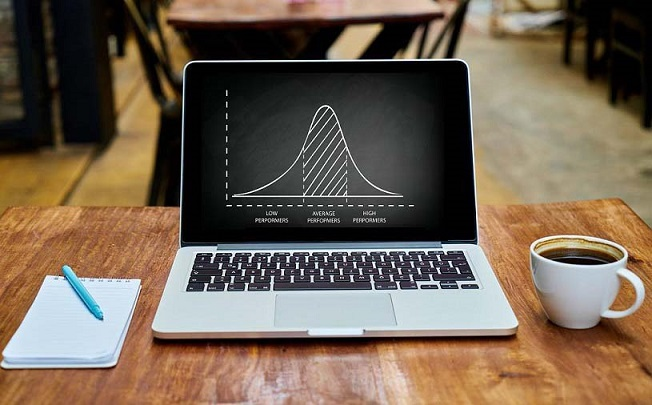
The Normal Distribution Curve
A variable or data set is said to be "normally distributed", if its distribution has the shape of a normal curve (Figure 1). The normal curve is very important in statistics, as well as in data analysis, because its essential for understanding and calculations of many inferential statistical tests such as t-test and Analysis of Variance (ANOVA).
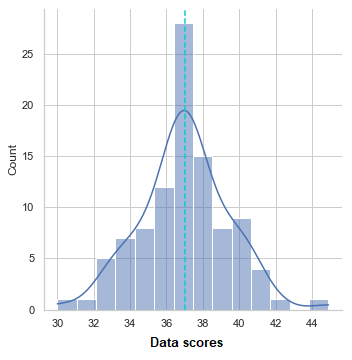
Figure 1: Normal distribution plot of a data sample.
The normal distribution curve is a bell-shaped curve centered at the mean (symmetric). Its mean equals 0; and the standard deviation equals 1. In addition, the total area under the normal curve equals 1, as shown in Figure 2.

Figure 2: The normal curve and its properties
In real research world, a data distribution is unlikely to have exactly the shape of a normal curve. If the distribution is shaped almost like a normal curve, we usually say that the variable is an approximately normally distributed.
The Empirical Rule
If the distribution of the data set is approximately bell-shaped; that are not too skewed, we can apply the empirical rule (Figure 3), which implies the following:
Approximately 68% of the data values lie within 1 standard deviation (σ) on each side of the mean.
Approximately 95% of the data values lie within 2 standard deviations on each side of the mean.
Approximately 99% of the data values lie within 3 standard deviations on each side of the mean.
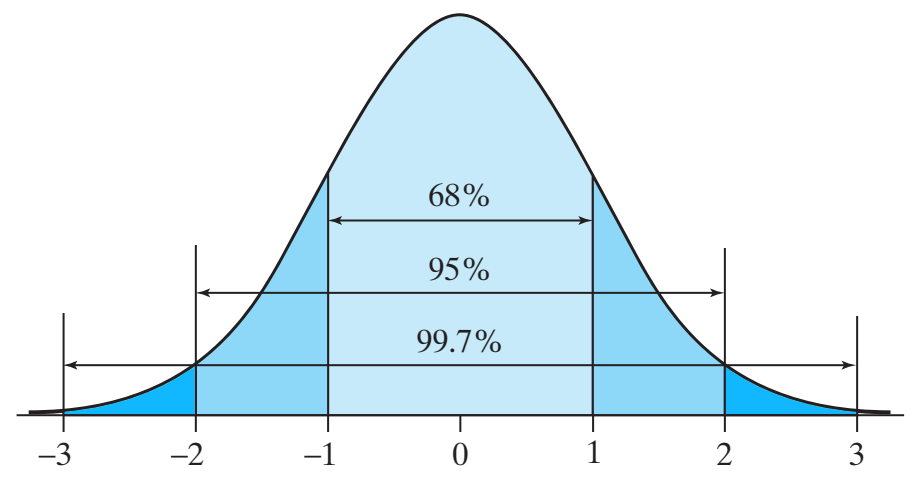
Figure 3: Areas under normal curve
Standardized Normally Distributed Variable
The empirical rule is very useful because by defining the areas under the standard normal curve, we can then use it as a scale to find areas under our normally distributed data curve. We do this by standardizing. In this context, standardizing means "transforming" every normal distribution into one particular normal distribution, known as the standardized normally distributed variable (z-score). This transformation can be performed by the following formula:

In other words, we determine areas under our data normal curve by transforming data score of our data into z-score distribution. Consequently, we can find the percentage (area) of all possible observations that lie within any specific range, as shown in Figure 4.
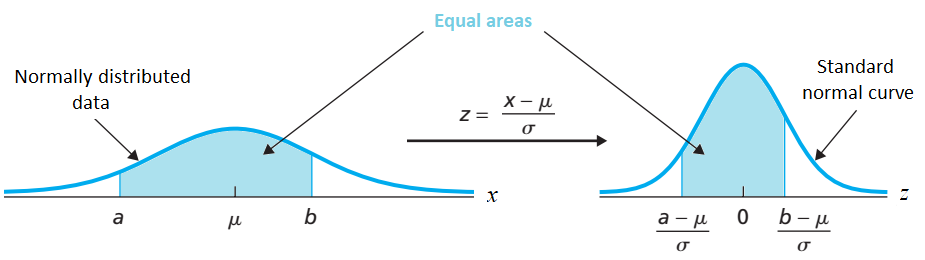
Figure 4: Illustration and formula for transforming raw scores into a z-scores.
We can do such calculations manually. However, researchers or analysts often do such calculations using one of many available statistical analysis software (SPSS, and SAS) or programming languages such (R and Python). For more details and calculations, we provide some useful resources listed in the Reference section at the end of this article.
To understand data analysis results and their interpretation, what we really need to understand is the z-alpha (zα). The z-alpha is simply the z-score that has an area to the right under the standard normal curve, as shown in Figure 5.
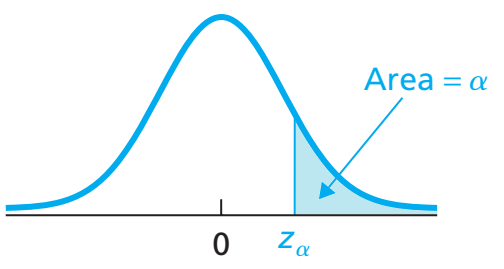
Figure 5: The alpha area under the normal curve which is above z-alpha.
For most researches hypothesis tests, we determine the confidence or significance level at 95% level (alpha = 0.05) or 99% level (alpha = 0.01).

Figure 6: The area under the normal curve when z-alpha at 0.05 level.
The z0.05 value is found in a table known as standard normal distribution table or z table. However, the alpha value depends on the distribution. In statistics there are other distributions to use, other than z-distribution, such as t-distribution for t-test and F-distribution for ANOVA, which has particular normal curve standards and calculations.
References
Heiman, G. W. (2011). Basic Statistics for the Behavioral Sciences (6th ed.). USA: Cengage Learning.
Samuels, M. L., Witmer, J. A., & Schaffner, A. (2012). Statistics for the Life Sciences (4th ed.): Pearson Education, Inc.
Weiss, N. A., & Weiss, C. A. (2012). Introductory Statistics (9th ed.): Pearson Education, Inc.
Mendenhall, W. M., & Sincich, T. L. (2016). Statistics for Engineering and the Sciences Student Solutions Manual (6th ed.). USA: Taylor & Francis Group, LLC.
Commenti