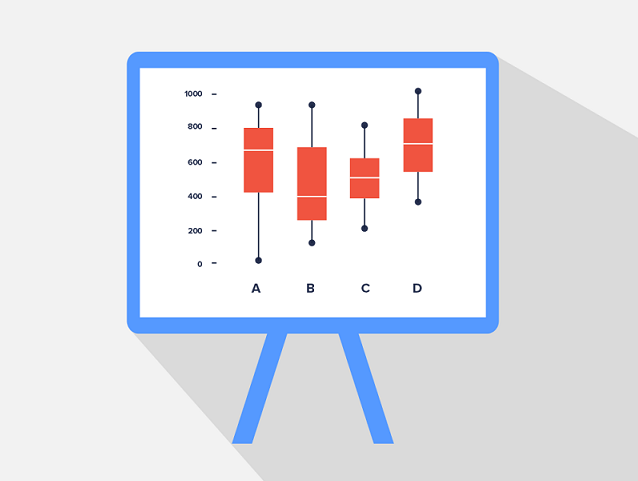
Table of Contents
In the previous article, we have focused on descriptive statistics such as mean, median and standard deviation to measure center tendency and variation. We now examine several descriptive measures based on percentiles.
Unlike the mean and standard deviation, descriptive measures based on percentiles or quartiles are resistant — this means that they are NOT sensitive to the influence of a few extreme observations (outliers). Therefore, descriptive measures based on quartiles are often preferred over those based on the mean and standard deviation.
The Five-number Summary
The five-number summary are points that represents five numbers derived from the data; the smallest data value (Min), the first quartile (Q1), the median (Q2), the third quartile (Q3), and the largest data value (Max).
These five numbers usually are represented in a boxplot (Figure 1), also known as box-whisker plots, which is a graphical representation based on these five-number summary.
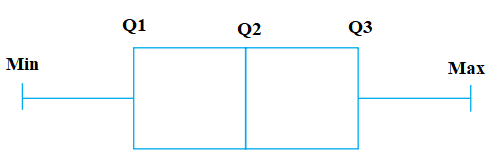
Figure 1: Boxplot illustration
Min and Max
The min is simply the lowest (minimum) data value, while the max is the highest (maximum) data value. It is easiest to determine the min and max if the data are ordered from lowest to highest.
Quartiles
As shown in Figure 2, the quartiles divide the sorted data set into four quarters using three points or values; the lower quartile (Q1), the median (Q2), and the upper quartile (Q3):
Q1 is the value that falls at the middle of the first 50% (lower half) of the data set.
Q2 is the median, which is the value that falls exactly at the middle of the data set.
Q3 is the value that falls at the middle of the second 50% (upper half) of the data set.
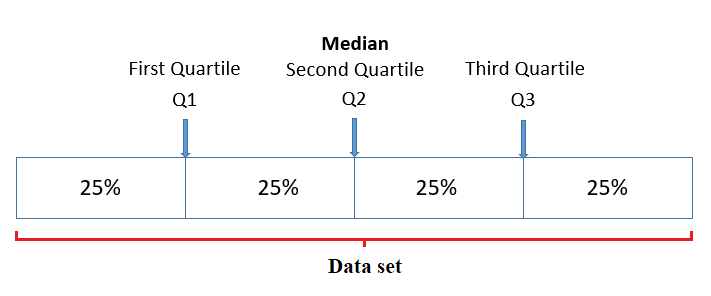
Figure 2: Illustration of the quartiles locations within a data set.
Example
The DDT_conc data set contains the poisonous DDT concentration in three fish species. We want to represent the data of the two fish species in a boxplot to visualize and compare the DDT concentration of each species.
We can detect descriptive statistics and visualize data using many software (e.g. SAS, and SPSS) or programming languages such as Python or R. In this example we used Python code (DDT-Descriptive) to obtain descriptive statistics and visualization.
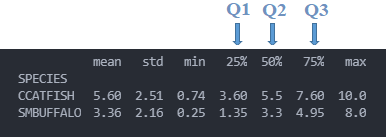

Figure 3: Boxplot and descriptive statistics obtained by Python.
Results presented in Figure 3 indicated that Catfish species has higher DDT concentrations than those observed in Buffalo species. Also, Buffalo species middle box (Q1, Q2, and Q3) are shifted toward the lower limit which means that most Buffalo species data values are within the lower DDT concentrations compared to Catfish data which slightly shifted toward the upper limit.
Outliers
Outliers are observations that fall outside the overall pattern of the data. It may be due to a measurement or recording error, an observation from a different population, or an unusual extreme observation. Sometimes one or two outliers can have a big impact on the data analysis results and consequently on the conclusions of the study.
For detecting observations that may be outliers, we can use quartiles and the IQR to identify potential outliers. To do so, we first define the lower limit and the upper limit of a data set:
Lower limit = Q1 - 1.5 · IQR
Upper limit = Q3 + 1.5 · IQR
The observations that lie below the lower limit or above the upper limit are potential outliers (Figure 4). To determine whether a potential outlier is truly an outlier, you should conduct data analysis techniques or constructing a boxplot, stem-and-leaf diagram, or other appropriate graphics.
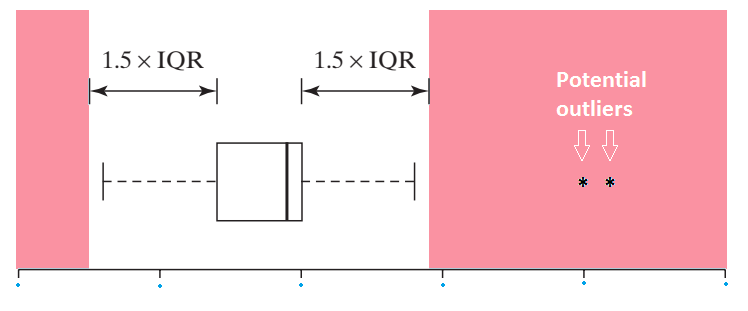
Figure 4: A boxplot illustration shows upper and lower limit area (in red), and potential outliers fall within this area.
References
Heiman, G. W. (2011). Basic Statistics for the Behavioral Sciences (6th ed.). USA: Cengage Learning.
Mendenhall, W. M., & Sincich, T. L. (2016). Statistics for Engineering and the Sciences Student Solutions Manual (6th ed.). USA: Taylor & Francis Group, LLC.
Samuels, M. L., Witmer, J. A., & Schaffner, A. (2012). Statistics for the Life Sciences (4th ed.): Pearson Education, Inc.
Weiss, N. A., & Weiss, C. A. (2012). Introductory Statistics (9th ed.): Pearson Education, Inc.
Comments